Can AI Replace Human Editors and Proof-Readers?
Five Reasons why They Won’t Anytime Soon
Leider ist diese Seite nur auf Englisch verfügbar. Der Inhalt wird unten in dieser verfügbaren Sprache angezeigt.
Contents
- 1. How do LLMs Work?
- 2. Why LLMs Won't Replace Human Editors for Some Time
- 2.1 Reason 1: Lack of Reliability due to the LLM's Inherent Inability to Truly Comprehend Text and Understand Figures and Tables
- 2.2 Reason 2: Difficulties With Texts Written by Non-Native Speakers
- 2.3 Reason 3: Inability to Understand the Complexity of Scientific Language and the Importance of Nuance and Audience Appropriateness
- 2.4 Reason 4: LLMs are Blind to Formatting and Only Process Short Portions of Text at a Time
- 2.5 Reason 5: Incapacity for Critical Thinking and Ethical Considerations
- 3. Conclusions
- 4. References
First posted: 18 June 2024 — Updated: 22 January 2025
Cite as: "XpertScientific. Can AI Replace Human Editors and Proof-Readers? Five Reasons why They Won’t Anytime Soon. January 2025. Online resource."
In recent years, artificial intelligence (AI) has made significant progress, particularly in natural language processing (NLP), with tools like ChatGPT demonstrating impressive abilities in generating text, answering questions, and even performing tasks such as editing and proofreading. These developments have sparked discussions about the potential for AI to replace human editors, proof-readers, and even authors. However, this prospect has also generated unease, particularly within the scientific community, where the written word is held to exceptionally high standards.
As a professional editing service, we were naturally curious about the editing and proofreading capabilities of current AI models. To explore their potential, we conducted extensive tests using texts with varying English proficiency and from various scientific disciplines. This article summarizes our findings, highlighting both the strengths of existing Large Language Models (LLMs) and their significant limitations that currently prevent them from fully replacing human expertise. These limitations are indeed numerous and, at the time of writing, include:
- "Hallucinations" (fabrication of information) and an inherent inability to fact-check
- An inability to truly comprehend language, which is particularly problematic when editing texts written by non-native speakers
- An inherent limitation in their ability to interpret figures and tables
- Difficulty in grasping the complexity of scientific language and the importance of nuance and audience appropriateness
- Blindness to formatting (cannot distinguish between normal text, headings, table content, etc.)
- Limited scope of analysis, restricted to page-by-page review without a full overview of the entire document
- Inability to engage in critical thinking
- Cannot work within the rules of style guides
Before discussing these issues in more detail, let us first examine how LLMs like ChatGPT construct sentences and process language.
How do LLMs Work?
LLMs like ChatGPT or Gemini operate by predicting the next word in a sentence based on the context provided by previous words, using a technique called “transformer architecture”. Trained on vast datasets containing text from books, articles, websites, and other sources, these models learn patterns in language, including grammar, syntax, and even some aspects of meaning and style. When constructing sentences and paragraphs, LLMs do not "understand" language in the same way humans do; rather, they generate text by selecting words that statistically fit the context based on the vast amounts of data that was used to train them.1,2,3
Furthermore, AI language models do not "read" documents in the way a human would. They process text in segments (like paragraphs or pages), i.e., they have a limited-size internal context window and cannot maintain an overview of long documents in their entirety. LLMs therefore rely on the context provided in the immediate text and do not retain information from previous sections of the document. This means that LLMs do not have a global understanding of an entire document at once but rather work with portions of text at a time.1,2,3
Despite these limitations, LLMs still manage to produce output that sounds natural and convincing. However, this is based purely on learned patterns and correlations rather than true comprehension or reasoning, leading to text that may be grammatically correct and contextually appropriate, but not necessarily factually accurate or logically valid. This brings us to our first item on the above list, the lack of reliability.
Take Home Messages
- LLMs are statistical models that lack true language comprehension and reasoning abilities.
- LLMs operate on isolated text segments without the capability to assess the entire document all at once.
Why LLMs Won't Replace Human Editors for Some Time
Lack of Reliability due to the LLM's Inherent Inability to Truly Comprehend Text and Understand Figures and Tables
Veracity is the cornerstone of scientific writing, where the accuracy and truthfulness of information are paramount. In scientific research, the integrity of arguments, data, and conclusions is critical not only for advancing knowledge but also for maintaining trust within the scientific community and the public. LLMs like ChatGPT, despite their advanced language processing abilities, currently lack the capability to verify the truthfulness of the information they generate or edit. Although they excel at generating contextually appropriate and linguistically sophisticated text, LLMs rely on pattern recognition rather than comprehension which leads to the risk of them introducing unrelated, factually incorrect, or completely fabricated content – a phenomenon known as "hallucinations", "confabulations" or, more simply, "bullshit".1-12 As these hallucinations are often obscured by well-crafted sentences, the underlying falsehoods can be difficult to spot, which has led to considerable embarrassment.13,14 Moreover, a comprehensive study assessing the performance of ChatGPT (3.5 and 4) and Google's Bard (now Gemini) to produce references in the context of scientific writing found that all LLMs failed to retrieve any relevant papers, with precision rates ranging from 13.4% for ChatGPT4 to 0% for Bard.15
Several authors have attempted to quantify truthfulness by simply counting the hallucinations produced by different models, which has resulted in a Hallucination Vulnerability Index16 and hallucination leaderboards.17,18 Using the very simple test case of tasking LLMs to summarize a given text passage — a closed situation which makes it easy to count hallucinations — Vectara found that for some LLMs up to 30% of the summarized information was completely fabricated, i.e., not contained in the original text passage. Even commonly used LLMs like Claude 2 (17.4%), Lama 2 13B (10.5%), and Google's Gemini 1.5 Pro (9.1%) performed rather poorly (Fig. 1).17 Even the latest iterations of OpenAI’s o1-mini and Google's currently experimental Gemini 2.0 Flash still yield hallucination rates of 1.3-1.4%, which seem surprisingly high considering the simplicity and closed nature of the task. Considering that most tasks put to LLMs are more complex than summarizing a short text passage, these findings should be considered baselines or best-case scenarios as more complex tasks may result in considerably higher hallucinations rates. This also serves as a reminder that although technological progress is reducing the frequency of hallucinations, they are not expected to ever disappear entirely as long as we continue to use statistical models that lack comprehension and logical reasoning capabilities.12
Furthermore, LLMs (as well as their multimodal extensions - MLLMs) are inherently limited in their ability to interpret figures and tables as they lack (i) training to comprehend the often intricate visual layout of scientific images, (ii) the reasoning capabilities needed to make subtle inferences from complex figures and datasets, and (iii) specialized knowledge and the high degree of contextual understanding required for such a task. In terms of editing, this limitation means that (M)LLMs are unable to assess whether the accompanying text provides a correct and adequate description of the corresponding figures and tables. This issue is especially pertinent in manuscripts written by non-native speakers. As a professional editing service, we often find that the Results section requires significantly more editing and correction than other parts of the manuscript. However, when we tested several models and tasked them to edit the Results sections of various manuscripts, we often found that their suggested revisions led to complete misrepresentations and even contradictions with the results presented in figures or tables.
Lastly, LLMs lack the capacity to assess the logical validity of arguments or to verify the consistency of data and conclusions presented within a manuscript. They are unable to cross-check whether results in one part of a document match those shown elsewhere (e.g., in a different paragraph, figure, or table) or align with established scientific principles. This limitation stems from the fact that LLMs, based on transformer architectures, are not designed for quantitative reasoning or to be Turing complete (unless enhanced with memory augmentation).19 Their primary function is to generate and understand text that mimics human language. For example, posing the same quantitative question to ChatGPT on different occasions (i.e., during different sessions) often yields varying responses. In science we do not want to approximate the answer to a problem if the solution is known or if it can be calculated unambiguously. This limitation underscores the indispensable role of human editors, who will critically evaluate the veracity of scientific content and spot logical inconsistencies, both within a sentence/paragraph and between distant sections of a large document. This is essential for preserving the credibility and reliability of scientific literature.
Take Home Messages
- As statistical models, LLMs are incapable of fact-checking or of comprehending text in the same way humans do, which results in them fabricating false and unsubstantiated content ("hallucinations").
- LLMs are essentially blind to figures and tables and therefore unable to provide reliable descriptions or ensure that accompanying text provides an adequate and accurate representation of results presented in graphical or tabular format.
- In scientific writing, where accuracy and truthfulness are paramount, hallucinations or approximations of the truth are simply unacceptable.
Difficulties with Texts Written by Non-Native Speakers
Scientists for whom English is a second language (ESL) often encounter substantial challenges when writing academic texts, as they must navigate not only the complexities of science but also the subtleties of English grammar, syntax, and vocabulary. In our professional editing service, we frequently observe issues where unclear phrasing can obscure the intended meaning. Sentences may become difficult to comprehend due to the presence of numerous grammatical errors, missing essential words or prepositions, or the incorrect use of specialized jargon. These issues can lead to awkward or ambiguous phrasing, incorrect usage of scientific terminology, and a lack of precision in conveying complex ideas. In this context, the inherent inability of LLMs to truly comprehend language becomes particularly problematic.
While both AI and human readers may occasionally struggle with interpretation, an experienced human editor, especially one with a background in the relevant scientific field, is far better equipped to infer the author's intent. Although AI tools can provide basic grammar and style corrections, they often struggle with more complex issues, particularly when sentences are unclear or when specialized terminology is used incorrectly. In our extensive experimentation with LLMs, we have consistently found that the more unclear a sentence and the more substantial the required corrections, the more likely AI is to misunderstand the intended meaning and produce corrections that are contextually inappropriate, incorrect (regarding the author's intended meaning), factually wrong (regarding established scientific truths), or simply failing to address deeper issues in clarity and accuracy. Consequently, especially for texts written by ESL authors, only a human editor with the pertinent scientific expertise will be able to accurately rephrase and clarify such texts, ensuring that the scientific message is conveyed correctly and effectively, something that AI cannot reliably achieve.
Take Home Messages
- Due to their inability to truly comprehend language, LLMs particularly struggle with texts authored by non-native English speakers.
- LLMs often misinterpret sentences that are grammatically incorrect or misuse terminology, leading to contextually inappropriate or factually incorrect corrections.
Inability to Understand the Complexity of Scientific Language and the Importance of Nuance and Audience Appropriateness
Scientific writing is characterized by its precision, technical terminology, and the need for clear, unbiased communication of complex ideas. While AI systems like ChatGPT can process and generate text based on patterns in large datasets, they struggle with the nuanced understanding required to accurately edit or proofread scientific manuscripts. We still remember how Google's chatbot Bard made history during its product launch demonstration in 2023 when it famously suggested that NASA's James Webb Space Telescope "took the very first pictures of a planet outside of our own solar system",20 which is of course incorrect since the Very Large Telescope in Chile did so first. Bard's error was based on it misunderstanding a statement it had seen on NASA's website which read "For the first time, astronomers have used NASA’s James Webb Space Telescope to take a direct image of a planet outside our solar system",21 illustrating how LLMs struggle with subtleties of language.
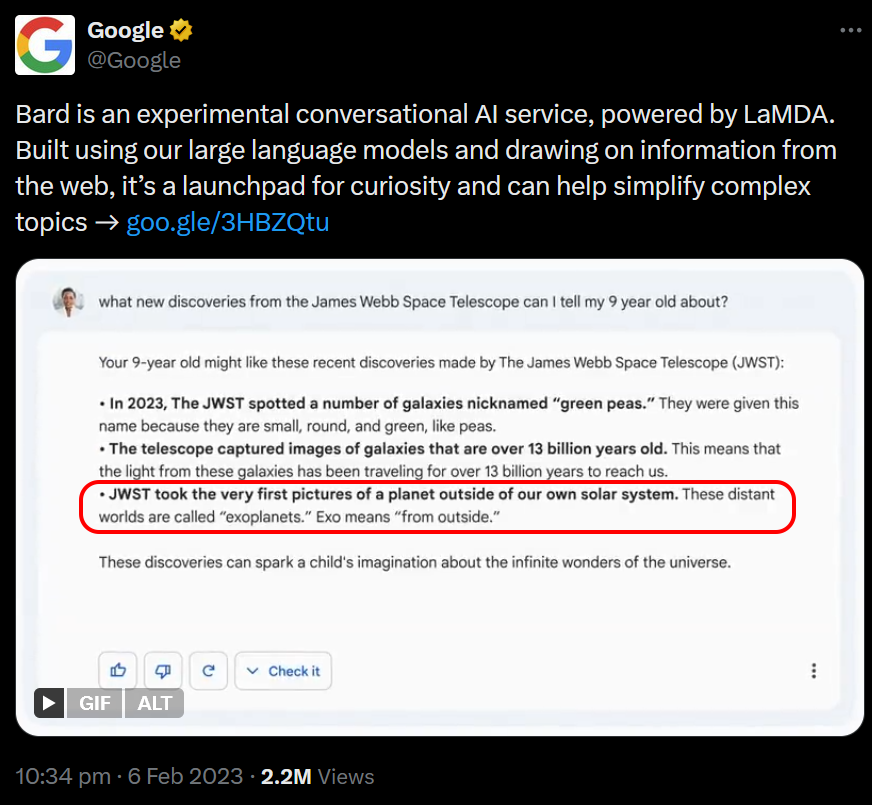
In addition, scientific language often involves the use of specialized jargon, acronyms, and terminology, as well as a particular "academic tone", that can vary significantly across disciplines. A human editor, particularly one with expertise in the relevant field, possesses the ability to recognize subtle errors in terminology, ensure consistency, and confirm that the language used adheres to the specific conventions of the discipline. Furthermore, scientific writing is not merely about conveying information; it is also about engaging in a dialogue with the scientific community. This requires a nuanced approach to communication, where the choice of words, the structure of sentences, and the presentation of data all play a role in how the manuscript is received and interpreted. Human editors are skilled in identifying and addressing these nuances, ensuring that the manuscript not only communicates its findings effectively but also aligns with the expectations of the scientific community. For instance, when dealing with statements of uncertainty or hypotheses, human editors can guide the author in using language that accurately reflects the degree of certainty or speculation involved. They can also help in crafting sections of the manuscript, such as the introduction or conclusion, to ensure that they adequately frame the research question and highlight the significance of the findings. AI, on the other hand, may generate text that is technically correct but lacks the subtlety required to navigate the complexities of scientific discourse.
Lastly, scientific manuscripts are carefully constructed arguments designed to communicate new findings to a specific audience, which may include peer reviewers, fellow researchers, or the broader scientific community. The success of a manuscript often hinges on how well it is tailored to its intended audience, and this requires a profound understanding of both the content and the context in which it will be received. Human editors bring a level of contextual awareness that AI currently lacks. They can discern whether the tone, style, and level of detail are appropriate for the target readership, whether it’s a high-impact journal or a specialized conference proceeding. Moreover, scientific writing often requires the integration of various types of information, such as data interpretation, methodological details, and theoretical implications. Human editors can evaluate the logical flow of arguments, identify potential gaps in reasoning, and suggest improvements that enhance the clarity and persuasiveness of the manuscript. AI, while capable of generating coherent text, often lacks the ability to critically assess the broader context in which a scientific argument is presented and may miss opportunities to strengthen the manuscript’s overall impact.
Take Home Messages
- Only human editors with subject-matter expertise can navigate specialized terminology, academic tone, and the subtleties of discipline-specific conventions.
- AI lacks the understanding of presentation and nuanced language required to effectively communicate scientific findings and meet the expectations of the scientific community.
- AI lacks the contextual and audience awareness needed to ensure that tone, style, and level of detail are appropriate for the target readership.
LLMs are Blind to Formatting and Only Process Short Portions of Text at a Time
Most LLMs lack the ability to recognize formatting distinctions, rendering them incapable of differentiating between standard text, headings, table contents, or captions. For instance, if you have a list of bullet points, each introduced by a bolded heading, a human editor can easily identify these elements and edit them appropriately. However, most LLMs fail to recognize such elements as headings and may attempt to convert them into complete sentences by unnecessarily adding verbs and prepositions (elements often omitted in headings), or they might even delete the headings entirely. This limitation in processing formatting also makes LLMs unsuitable for adhering to specific style guides, such as those required by a target journal's author instructions.
Additionally, LLMs do not have internal memory (a pre-requisite for true intelligence), and therefore process text in short, isolated segments. When an AI like ChatGPT edits a document, it treats each page — or even each paragraph — independently, rather than as part of a cohesive whole. This approach is akin to assigning each page of a manuscript to a different human editor, leading to a disjointed mix of editing styles and making it impossible to detect inconsistencies that span the entire document, such as variations in terminology and spelling or logical incoherences.
Take Home Messages
- AI is blind to formatting distinctions, potentially resulting in improper corrections that violate prescribed style guides.
- LLMs, with no internal memory, can only process short, isolated text segments, often overlooking or introducing logical contradictions and inconsistencies in style and terminology.
Incapacity for Critical Thinking and Ethical Considerations
One of the most significant limitations of AI in scientific editing and proofreading is its inability to engage in critical thinking. Editing a scientific manuscript often involves evaluating the logical coherence of arguments, identifying potential biases or ethical concerns, and ensuring that the conclusions are supported by the data presented. Unlike AI, human editors are adept at detecting flaws in reasoning, such as overgeneralizations, unwarranted assumptions, or unsupported claims, and can provide feedback that helps authors strengthen their arguments and align their work with the standards of scientific rigour.
Ethical considerations are also paramount in scientific writing. Issues such as plagiarism, data fabrication, and inappropriate authorship practices require careful scrutiny, and human editors play a crucial role in identifying and addressing these concerns. While AI can be trained to recognize certain patterns associated with ethical issues, it lacks the judgment and contextual awareness needed to fully assess the ethical implications of a manuscript. Human editors, with their experience and understanding of the ethical standards in scientific research, are better equipped to guide authors in maintaining the integrity of their work.
Take Home Messages
- LLMs cannot reason, rendering them unable to detect logically incoherent arguments, potential biases, ethical concerns, or unwarranted assumptions, claims, or conclusions.
- LLMs lack the judgment and contextual awareness necessary to fully assess ethical issues, such as plagiarism, data fabrication, and inappropriate authorship practices.
Conclusions
While AI tools like ChatGPT have demonstrated significant potential in assisting with tasks related to language processing, they are not poised to replace human editors and proof-readers in the scientific domain anytime soon. The absolute need for veracity in scientific writing, the complexity of scientific language, the need for contextual understanding, the nuances of scientific communication, and the importance of critical thinking and ethical considerations are all areas where human expertise remains indispensable. For the foreseeable future, human editors will continue to play a crucial role in ensuring that scientific manuscripts are not only grammatically correct but also clear, logically coherent, and ethically sound. While AI can serve as a valuable tool in the editing process, it is unlikely to fully replicate the depth of understanding and the critical judgment that human editors bring to the task.
References
- OpenAI. Introducing ChatGPT. November 2022. Online resource
- OpenAI et al. GPT-4 Technical Report. arXiv. 2023; arXiv:2303.08774. DOI: 10.48550/arXiv.2303.08774
- Google. Gemini Apps FAQ. November 2023. Online resource
- Emsley R. ChatGPT: these are not hallucinations – they’re fabrications and falsifications. Schizophr. 2023; 9:52 DOI: 10.1038/s41537-023-00379-4
- Hicks MT, Humphries J, Slater J. ChatGPT is bullshit. Ethics Inf Technol. 2024; 26:38. DOI: 10.1007/s10676-024-09775-5
- Zhou L, Schellaert W, Martínez-Plumed F, et al. Larger and more instructable language models become less reliable. Nature. 2024. DOI: 10.1038/s41586-024-07930-y
- Lee P, Bubeck S, Petro J. Benefits, Limits, and Risks of GPT-4 as an AI Chatbot for Medicine. N Engl J Med. 2023; 388(13):1233-1239. DOI: 10.1056/NEJMsr2214184
- Alkaissi H, McFarlane SI. Artificial Hallucinations in ChatGPT: Implications in Scientific Writing. Cureus 2023; 15(2):e35179. DOI:10.7759/cureus.35179
- Dolan EW. ChatGPT hallucinates fake but plausible scientific citations at a staggering rate, study finds. Artificial Intelligence. 2024. Online resource
- Xu Z, Jain S, Kankanhalli M. Hallucination is Inevitable: An Innate Limitation of Large Language Models. arXiv. 2024; arXiv:2401.11817. DOI: 10.48550/arXiv.2401.11817
- Huang L, Yu W, Ma W, Zhong W, Feng Z, et al. A Survey on Hallucination in Large Language Models: Principles, Taxonomy, Challenges, and Open Questions. arXiv. November 2023; arXiv:2311.05232. DOI: 10.48550/arXiv.2311.05232
- Jones, N. AI hallucinations can’t be stopped — but these techniques can limit their damage. Nature. 2025; 637:778-780. DOI: 10.1038/d41586-025-00068-5
- Sato M, Roth E. CNET found errors in more than half of its AI-written stories. The Verge 2023. Online resource
- Weiser B. Here’s What Happens When Your Lawyer Uses ChatGPT. The New York Times 27 May 2023. Online resource
- Chelli M, Descamps J, Lavoué V, Trojani C, Azar M, Deckert M, Raynier JL, Clowez G, Boileau P, Ruetsch-Chelli C. Hallucination Rates and Reference Accuracy of ChatGPT and Bard for Systematic Reviews: Comparative Analysis. J Med Internet Res. 2024; 26:e53164. DOI: 10.2196/53164
- Rawte V, et al. The Troubling Emergence of Hallucination in Large Language Models -- An Extensive Definition, Quantification, and Prescriptive Remediations. arXiv. October 2023; arXiv:2310.04988. DOI: 10.48550/arXiv.2310.04988.
- Hong G, et al. The Hallucinations Leaderboard - An Open Effort to Measure Hallucinations in Large Language Models. arXiv. May 2024; arXiv:2404.05904. DOI: 10.48550/arXiv.2404.05904.
- Jain K, Kazi S, Mendelevitch O. Correcting Hallucinations in Large Language Models. Vectara Sept 2024. Online resource
- Schuurmans D. Memory Augmented Large Language Models are Computationally Universal. arXiv. Jan 2023; arXiv:2301.04589. DOI: 10.48550/arXiv.2301.04589
- Google [@Google]. (2023, 6 Feb 2023). Bard is an experimental conversational AI service, powered by LaMDA. Built using our large language models and drawing on information from the web, it’s a launchpad for curiosity and can help simplify complex topics. X (formerly Twitter). https://x.com/Google/status/1622710355775393793
- Fisher A. NASA’s Webb Takes Its First-Ever Direct Image of Distant World. NASA Sept 2022. Online resource